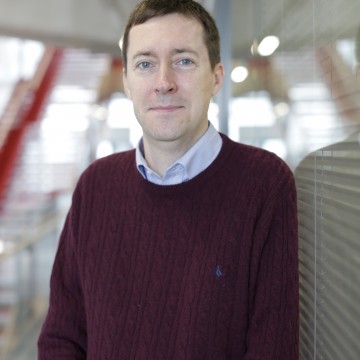
Declan O'Regan
Group Members
Funding
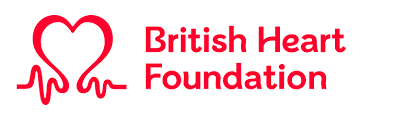

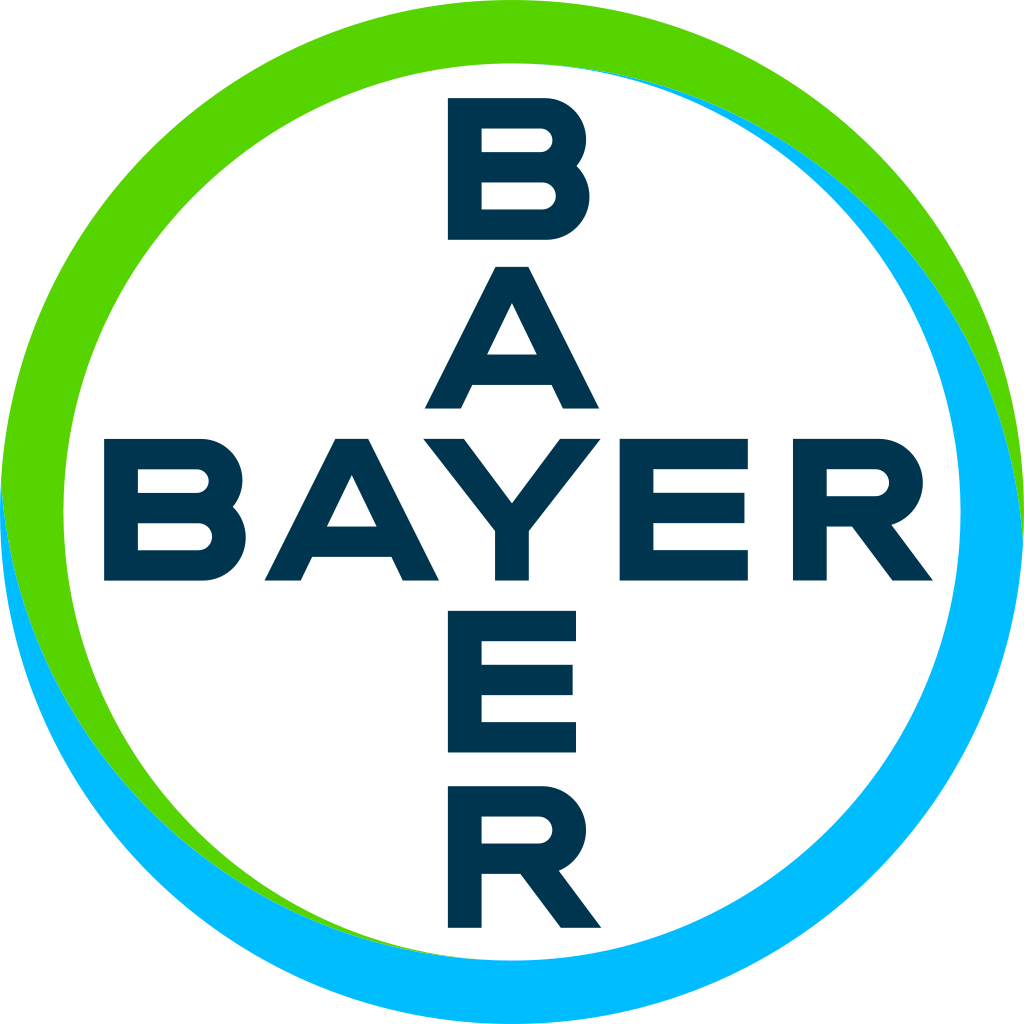
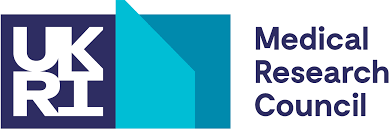

“We explore the mechanisms underlying heart function using machine learning to analyse cardiac motion for predicting patient outcomes, discovering potential therapeutic targets and identifying genetic risk factors.”
Research areas:
Image segmentation and motion analysis: Techniques for vision-based motion analysis aim to understand the behaviour of moving objects in image sequences. In this domain, deep-learning architectures have achieved a wide range of competencies for object tracking, action recognition and semantic segmentation. We are developing novel deep learning techniques for segmentation, motion analysis and registration of diverse cardiac MRI datasets that are efficiently scalable to large populations. This work provides a framework for quantitatively phenotyping complex cardiac traits including function, geometry and tissue characteristics for classification and prediction tasks.
Automated adverse event prediction in heart disease: Current approaches for survival prediction rely on hand-crafted features that lack precision and are insensitive to the complex pathophysiology of heart failure. We are evaluating the use of deep learning algorithms to predict time-to-events from complex input data that include motion phenotypes, genetics and clinical variables. We are also exploring how to integrate irregularly sampled data and model competing risks in heart disease as a step towards the development of artificial reasoning systems for automated analysis and interpretation of clinical investigations to optimise management decisions.
Genotype-phenotype modelling: Substantial advances have been made in understanding the molecular basis and clinical manifestations of cardiomyopathy, however little is known about why disease expression and outcomes are so variable – manifesting as age of onset, severity of remodelling, and the cumulative lifetime burden of cardiovascular events. We are exploring whether task-driven clustering of high-dimensional data can optimally define cardiomyopathy classes by outcome and response to therapy compared to conventional risk groups. We are also attempting to understand the transition from health to disease by determining if pathogenic variants in cardiomyopathy-associated genes predispose to phenotypic adaptations in the general population and relatives of probands.
Identifying disease-modifying molecular pathways: We are investigating whether common polymorphisms and low-frequency missense variants in genes of interest contribute to a genetic predisposition to altered mechanical and tissue characteristics – using large-scale imaging and whole-exome sequencing in UK Biobank. These approaches harness the power of machine learning for high-throughput imaging-genetics research on large populations to prioritize genes for which pharmacologic agents may lower risk for disease.
Software – software and other tools developed by the group are available at https://github.com/UK-Digital-Heart-Project.
Machine learning cardiac motion analysis of patients diagnosed with right heart failure is used for automated survival prediction (DOI:10.1038/s42256-019-0019-2).
Selected Publications
Thanaj, M., Mielke, J., McGurk, K.A., Bai W., Savioli N., de Marvao A., V Meyer H., Zeng L., Sohler F., Lumbers R. T., Wilkins M. R., Ware J. S., Bender C., Rueckert D., MacNamara A., Freitag D. F., O’Regan D. P. (2022). Genetic and environmental determinants of diastolic heart function. Nat Cardiovasc Res 1, 361–371. https://doi.org/10.1038/s44161-022-00048-2
Meyer HV, Dawes TJW, Serrani M, Bai W, Tokarczuk P, Cai J, de Marvao A, Henry A, Lumbers RT, Gierten J, Thumberger T, Wittbrodt J, Ware JS, Rueckert D, Matthews PM, Prasad SK, Costantino ML, Cook SA, Birney E, O’Regan DP. (2020). Genetic and functional insights into the fractal structure of the heart. Nature, 584; 589–594.
Bai W, Suzuki H, Huang J, Francis C, Wang S, Tarroni G, Guitton F, Aung N, Fung K, Petersen SE, Piechnik SK, Neubauer S, Evangelou E, Dehghan A, O’Regan DP, Wilkins MR, Guo Y, Matthews PM, Rueckert D. (2020). A population-based phenome-wide association study of cardiac and aortic structure and function. Nature Medicine, https://doi.org/10.1038/s41591-020-1009-y.
Bello GA, Dawes TJW, Duan J, Biffi C, de Marvao A, Howard LSGE, Gibbs SR, Wilkins MR, Cook SA, Rueckert D, O’Regan DP. (2019). Deep learning cardiac motion analysis for human survival prediction. Nature Machine Intelligence, Vol:1, ISSN:2522-5839; 95-104
Dawes TJW, de Marvao A, Shi W, Rueckert D, Cook SA and O’Regan DP. (2019). Identifying the optimal regional predictor of right ventricular global function: a high-resolution three-dimensional cardiac magnetic resonance study. Anaesthesia. 74, 312-320. DOI:10.1111/anae.14494.
Dawes TJW, Cai J, Quinlan M, de Marvao A, Ostowski PJ, Tokarczuk PF, Watson GMJ, Wharton J, Howard LSGE, Gibbs SR, Cook SA, Wilkins MR, O’Regan DP. (2018). Fractal analysis of right ventricular trabeculae in pulmonary hypertension. Radiology, Vol:288, ISSN:0033-8419; 386-395
Biffi C, de Marvao A, Attard MI, Dawes TJW, Whiffin N, Bai W, Shi W, Francis C, Meyer H, Buchan R, Cook SA, Rueckert D, O’Regan DP. (2018). Three-dimensional cardiovascular imaging-genetics: a mass univariate framework. Bioinformatics. 34, 97-103. DOI:10.1093/bioinformatics/btx552.